Scientists in China and the United States say they have developed a new artificial intelligence (AI) model that could help overcome some major challenges to drug development and discovery.
The model, called ActFound, outperforms competing models while bypassing challenges to using machine learning in bioactivity prediction, according to a paper published in Nature Machine Intelligence.
“Bioactivity encompasses various properties of compounds, such as their interaction with targets, impact on biological systems and therapeutic effects,” said the researchers from Peking University, the University of Washington and AI tech firm INF Technology Shanghai.
The main challenges to using machine learning include limited data labelling and incompatibility between assays, the tests that measure the activity or potency of drugs.
The model not only outperforms competing AI models, but also functions as well as free-energy perturbation (FEP) – a traditional computational method.
Although FEP calculations have a high level of accuracy, the team warned that they “require extensive computational resources that are often not affordable for large-scale applications”.
Such methods often rely on hard-to-obtain, three-dimensional protein structures to run, which can only be obtained using expensive equipment and extensive laboratory procedures.
The team said ActFound could accurately function with fewer data points, offering an accurate and less costly alternative to FEP.
“Our promising results indicate that ActFound could be an effective bioactivity foundation model for various types of activities,” said Wang Sheng, corresponding author and assistant professor at the University of Washington.
China is home to a booming pharmaceutical industry, with the government investing heavily in research and development for innovative drugs.
Some companies have turned to AI to find potential drug targets in a bid to cut development time, with some products already undergoing clinical trials.
“Evaluating the bioactivity of compounds is central to drug discovery and development,” the team wrote in their paper.
Bioactivity prediction aims to predict values for compounds, helping scientists to identify potentially useful compounds from a large number of candidates while minimising time-consuming and expensive experiments.
But despite machine learning’s potential, some major challenges limit its adoption.
“Existing machine learning approaches have poor generalisability in bioactivity prediction due to the small number of compounds in each assay and incompatible measurements among assays,” the team wrote.
Foundation models, which are pre-trained on large data sets to generalise prediction for unlabelled data sets, are one way to bypass these issues.
ActFound was trained using 35,644 assays from a popular chemical database as well as 1.6 million experimentally measured bioactivities.
It also uses two machine learning methods: meta-learning and pairwise learning.
Meta-learning is a framework that allows a model to be optimised using limited labelled data to predict the properties of unmeasured compounds. A model trained with a large number of assays can be used to work within assays with limited data.
Meta-learning “is well suited for bioactivity prediction, because there is a severe shortage of unmeasured bioactivity data in many drug discovery projects due to the high costs of laboratory experiments,” Wang said.
Meanwhile, pairwise learning helps generalise the model. Instead of predicting potentially incompatible absolute values, it calculates the relative differences between compound pairs.
“Our intuition was that, although compounds from different assays may have different units, value ranges or measurement metrics, those within the same assay are comparable,” the team wrote.
“As far as we know, we were the first to combine meta-learning and pairwise learning in bioactivity prediction.”
ActFound was tested on six real-world bioactivity data sets, and was found to outperform nine competing models for in-domain prediction and performed strongly in cross-domain prediction.
The team also conducted a case study to see if their model could be used to predict the bioactivity of cancer drugs, and found it performed better than other models.
“Our promising results indicate that ActFound could be an effective bioactivity foundation model for compound bioactivity prediction, paving the way for machine-learning-based drug development and discovery,” the team said.
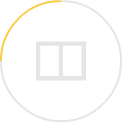